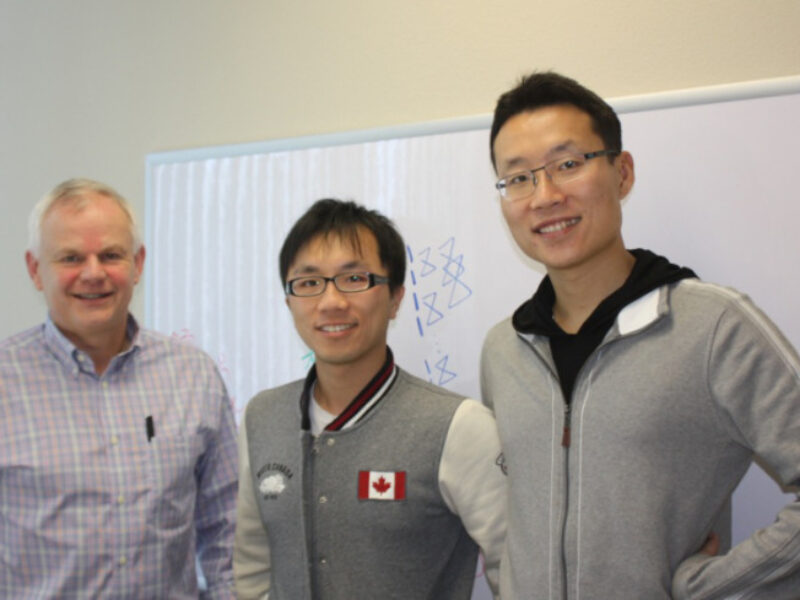
Interview – Flex Logix CEO Geoff Tate
FPGA chip maker Flex Logix is taking on industry giant Nvidia with a new chip for machine learning for vision systems. It has adapted its interconnect fabric for an application specific inference engine for machine learning at the edge using a 16nm process but is looking at the second generation on 7nm.
The chip is optimised for video images and large machine learning models, rather than being a general purpose AI chip, says Geoff Tate, CEO and co-founder of Flex Logic and former founder of Rambus and general manager of AMD’s processor business (above left with his co-founders).
“Our focus is on the edge, out in the real world, ultrasound systems, camera applications, autonomous vehicles, gene sequencing and automatic inspection,” said Tate.
“Other than autonomous vehicles, customers have a single sensor and bringing in rectangular ‘images’ with depth information. All these customers have a single model and they don’t necessarily care how other models run and finding a chip that will run it fast and cheap, and this is where we get application specific inference. They want more throughput and lower cost.”
Like competitor Blaize, the Flex Logix chip is a graph processor, relying on the compiler to allocate the resources of the chip to the AI model.
“Ultrasound or MRI use big models and large images,” he said. “The smallest is 0.5Mpixel up to 4Mpixel. We run the largest models – the weights are 62Mbytes of data and our customers want to run big images and don’t want to give up on precision.”
“You can’t run these models cost effectively on an FPGA – if you want to implement the entire model you need a very large FPGA and those are very expensive and every layer of the model has to be implemented. We discarded that idea a long time ago and we solve the problem by rapidly reconfiguring in microseconds.”
The chip is 54mm2 in 16nm TSMC process with a worst case thermal TDP envelope of 7 to 13W, making it eminently suitable for edge design. “We will be selling in a 21 x 21mm flip chip and as PCIe boards – there are systems such as gene sequencing or MRI that can have a server rack so its easier for them to integrate the technology by plugging in boards.”
The chip uses an array of 1 dimensional vector processing units that can be reconfigured in 4us to allow multiple layers of a neural network to be calculated. This gives a throughput/mm2 that is 3 to 18x more efficient than the GPU, says Tate, which will always be an advantage if chips are on the same process technology.
“The reason we pick this approach this gives the finest granularity,” he said. “It brings in a tensor in 64 cycles and does a 64 x 64 matrix multiple, shifting out the results, and the inputs and outputs can be connected programmably to other TPUs or memory. The processors are in tiles of 16, so we snap together into array and this implementation is 64 processors which is for tiles.”
“Reconfiguring at high speed is a matter of a cache memory which holds the configuration for the next layer and shift in in 4us. We also have a lot of SRAM hidden around the chip, in the TPU for the weight matrix for example which we call L0, L1 close to processor, L2 that is segmented into blocks that are individually addressable for the 64 x64 multipliers and then L3, which is about 1Mbit for configuration memory. We can always have a full bandwidth non blocked path through the processors to memory, that gives the high utilisation to memory,” he said.
This reconfiguration comes at minimal cost, he says. “Large models such as YOLOv3 [You Only Look Once] require upwards of 300bn MAC operations, with 3bn MAC operations per layer on average. That takes much longer than the reconfiguration time, accounting for 0.2 per cent of the total execution time,” he said.
“We think YOLOv3 will dominate the market but our architecture is reconfigurable for other operators such as 3D convolution in life sciences. Customers with high volume applications will be using a certain set of models and we will continue to add more operators,” he said.
The first PCI Express card, the X1P1, will have a single chip and cost $499, while the X1P4 car with four chips will give a throughput similar to Nvidia’s T4 card for half the price,” says tate. “We are not going to replace T4 in applications, we are looking to expand the market, we want to see much higher volumes.”
The first chip was deliberately on 16nm to get to market but will move to 7nm for higher performance, smaller size and cost and lower power. “[Design house] GUC did all the physical back end for the SOC parts of the chip but we use 180nm to 12nm for embedded FPGA so we have a lot of experience,” said Tate. “We have started the design work at 7nm and don’t see any issues.”
Related edge AI chip articles
- RISC-V BOOM FROM EDGE AI SAYS FACEBOOK’S CHIEF AI SCIENTIST
- AI CHIP ADDS MESH PROCESSING
- ALTRAN AND INTEL OPEN UP EDGE TELECOMS PLATFORM
- BLAIZE DETAILS ARCHITECTURE OF GSP CHIP
Other articles on eeNews Europe
- Multicore GPU aims at 3nm for data centre designs
- Xilinx teams for cloud-based open source Covid-19 X-ray classification
- Somos Semi deal boosts ST’s IoT roadmap
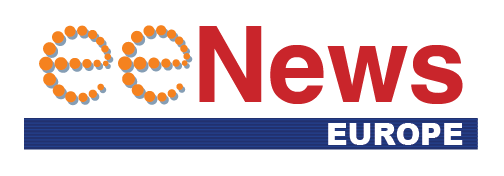